Ever since robots were conceived by science fiction writers, they captured the fascination of humans. And they gave rise to fears. How founded is a recent fear is that robots might soon displace humans in most jobs, thus creating a permanent underclass?
Sam Bowman from Adam Smith Institute, the British libertarian think tank writes: “Eventually someone may invent a robot that is better than humans at virtually any given job. It would be cheaper to produce and maintain and faster, smarter, and better at learning. But even until then, we will probably keep inventing robots that are better than a significant portion of the labour force at a job that they do.”
“If a significant portion of the workforce cannot produce more efficiently than a robot they will become permanently unemployed. Human labour is an input and markets are about outputs. There are lots of basically-useless inputs that the market does not use much and the time will probably come when the labour of a large number of human beings will be included in this category.”
I, on the contrary, would argue that the widespread belief, which approaches conventional wisdom, that robots are capable of permanently displacing human labour is based on a misunderstanding of what computers – and, thus, robots — actually do, when they are used to solve problems.
For most people, the functioning of computers is deeply mysterious. Thus, when computers achieve impressive results, like beating the world champion at chess, it creates an impression that they will soon overtake us in terms of intelligence. However, once we realize what robots actually do, it becomes clear that they are incapable of a genuine understanding.
After all, computers follow sets of coded instructions. Programmers might not be able to follow all logical implications of the programs they design and it doesn’t mean that a computer that produced unexpected results is an instance of artificial intelligence, like Skynet in the Terminator sequel, that it suddenly gained awareness and is about to decide that it should get rid of its masters.
The Chinese Room
In his famous thought experiment called “Chinese Room”, the philosopher John Searle convincingly demonstrated that even a human mindlessly following instructions does not qualify as understanding what she or he is doing.
In Searle’s hypothetical set-up, a human is put in a room separated from the outside world and given a set of instructions on how to respond to specific Chinese hieroglyphs with other Chinese hieroglyphs. But even if her instructions and performance succeed to convince some Chinese native-speakers outside of the room, communicating with her that way, that she understands Chinese, it is clear that she can be totally clueless about Chinese. In Searle’s words, she has “syntax but no semantics”.
Searle’s argument prompted a heated debate. One of the most popular objections was the model of the computer was misidentified: it cannot be identified solely with the subject of the experiment but has to include also the hieroglyph-handling instructions. To rebut this objection, it is enough to contemplate what would happen if the subject encountered a hieroglyph or combination of hieroglyphs for which there is no instruction. If the subject had a genuine grasp of Chinese, Chinese speakers outside could use other hieroglyphs to make her understand the meaning of the problematic combination. It is clear that the instructions will be of no help whatsoever.
In my opinion, the implication of this is that robots might never cope in a situation that was not taken into account by their programmers. There are jobs that involve reacting to genuinely new circumstances and thus cannot, in general, be automated. It is difficult to come up with a detailed list of such jobs, but even seemingly simple jobs may involve facing unexpected and high-stake situation. I personally witnessed such a situation in a bar in Aix-en-Provence where a violent brawl erupted outside of the bar and a part of the fighting group attempted to take refuge in the bar. The bartenders were expected to quickly decide what to do about it. They didn’t let the group in.
Neural networks, deep learning, and their limits
Supporters of the “permanent labour displacement” hypothesis may, however, still claim that handling uncommon, unpredictable situations is not essential for most jobs. Even if it is difficult to conclusively prove this point one way or the other, there is a potentially more important hurdle for robots if they were to replace human labour completely.
In order for a robot to execute a program, it must determine what are the relevant elements in place. If a robot performs the functions of a waiter, for example, it must recognize the objects it is handling, the clients’ requests, and so on. One of the most important recent innovations relevant to such recognition was the advent of neural network and the associated deep learning.
The precise ways in which neural networks recognize are multiple and highly technical, but they all share the same basic approach. A neural network is a computer program that has successive layers of weights assigned to inputs that it has to deal with and a procedure of optimizing them based on the examples on which it is “trained” in order to achieve the minimum recognition failure rate. In image recognition, inputs are data arrays derived from arrays of pixels, which constitute images. When confronted with an image, a neural network essentially assigns weights to its constitutive parts, based on its past trainings.
While they may be highly efficient in particular tasks, researchers have shown that neural networks function in a fundamentally different way from the way humans form concepts. Neural networks would sometimes misidentify images that they previously correctly identified because of small modifications imperceptible to humans. In addition, one can generate images that are deliberately meaningless a human eye but that are classified by neural networks as objects: guitars, bubbles, peacocks, etc. Since this appears to be an intrinsic feature, it is doubtful whether tinkering with increasingly sophisticated neural networks can bring the errors down to acceptable rates.
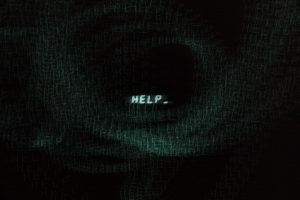
A message from artificial intelligence (photo: Michael Cordedda/Flickr)
Similarly, a recent study has demonstrated a profound problem with language recognition in a contest with chatbots based on deep-learning. The Winograd Schema Challenge asks computers to make sense of ambiguous sentences, which humans can still understand. Consider the following sentence: “The city councilmen refused the demonstrators a permit because they feared violence.” It is logically unclear who the word “they” refers to, yet for most humans, it is obvious because of the broader context and common-sense. The software programmes that entered the challenge were a little better than random at choosing the correct meaning of sentences.
Again, it appears that the problem here is intrinsic. The way that neural networks handle text is fundamentally different from genuine understanding, and no heroic coding effort seems to help.
Even though a robot will be more efficient than humans in routine tasks, the described deficiencies of the most advanced recognition techniques are crucial, because, in many contexts, gross errors that humans will never make may cause unacceptable damage.
Let us consider the example of waiters. Robots may be more efficient than humans in the sense that they won’t forget or misremember some of the ordered items, for instance. But if a robot misunderstands a clear request by a client not to put nuts into a dish because the client has a severe allergy, this may do irreparable harm both to the client’s health and to the establishment’s reputation.
All points considered, it seems more plausible that rather than displacing humans at work en masse, robots and humans will continue to be employed side-by-side, each specializing at the kind of things he, she, or it does better. Instead of a catastrophe envisaged by many, this could well allow people to work less and be more creative.
Daniil Gorbatenko is a PhD candidate in economics at Aix-Marseille University in Aix-en-Provence, France, a member of Students for Liberty Aix-Marseille. This piece was originally published on Medium.